This blog is a recap of, “Laying the Groundwork: How to Prepare for an AI Implementation” TheLoops fireside chat featuring Anthony Broomes-Barbee, Director of Customer Support at Energage.
The benefits of AI in customer experience are no longer theoretical—they’re measurable and real. CX leaders now recognize that driving efficiency doesn’t just reduce costs; it creates a better environment for agents and improves the customer experience overall.
In fact, 64% of Customer Service leaders plan to spend more time in 2025 mastering AI tools, often prompted by top-down pressure to modernize operations and meet rising expectations.
However at Energage, a unified SaaS platform that helps you build and brand your workplace as a top employer, the shift to AI didn’t come from the top. It started on the front lines.
Rather than waiting for executive mandates, Support management took initiative by proactively championing the launch of an AI Agent Copilot to solve persistent challenges. What makes this story even more compelling for others in the throws of AI adoption is how the Energage team was able to go live with their first use case in under 60 days.
In this blog, we’ll share the steps Anthony Broomes-Barbee and his colleagues took to get started, how he prepped their data and the key takeaways he offers for any team navigating similar challenges. If you’re thinking about how to introduce AI Agents into your own support workflows—without delays and an ability to validate ROI—this blog is for you.
Understanding Your Requirements—Vendor Selection & Strategy
Traditionally, the first step in evaluating any new technology anchors around use case and vendor selection. At first, Anthony thought a customer-facing chatbot was the obvious entry point. Not one to follow the crowd, he dug in a little deeper to understand where they had bottlenecks. What were the problems he and his team were trying to solve?
- They were always asking a lot of questions and knew they could surface information faster
- Agents were geographically dispersed, with a large portion of the team in South America
- Grammar, tone and clear communication were all things the team managed and oversaw manually
- That process alone could take anywhere from 60–140 minutes/day at minimum equating to anywhere from 5–11.5 hours/week per agent
With this in mind, chatbots were ruled out as the first use case. Instead, AI Agent Copilot became the focal point. Next, it came down to vendor selection. He defined clear requirements and success criteria for his support organization:
- Seamless integration with Salesforce and other existing support tools
- Chatbot capability for future self-service deployment
- Actionable analytics on knowledge-base gaps and ticket trends
- Continuous learning so the AI could train and refine itself with context on an ongoing basis
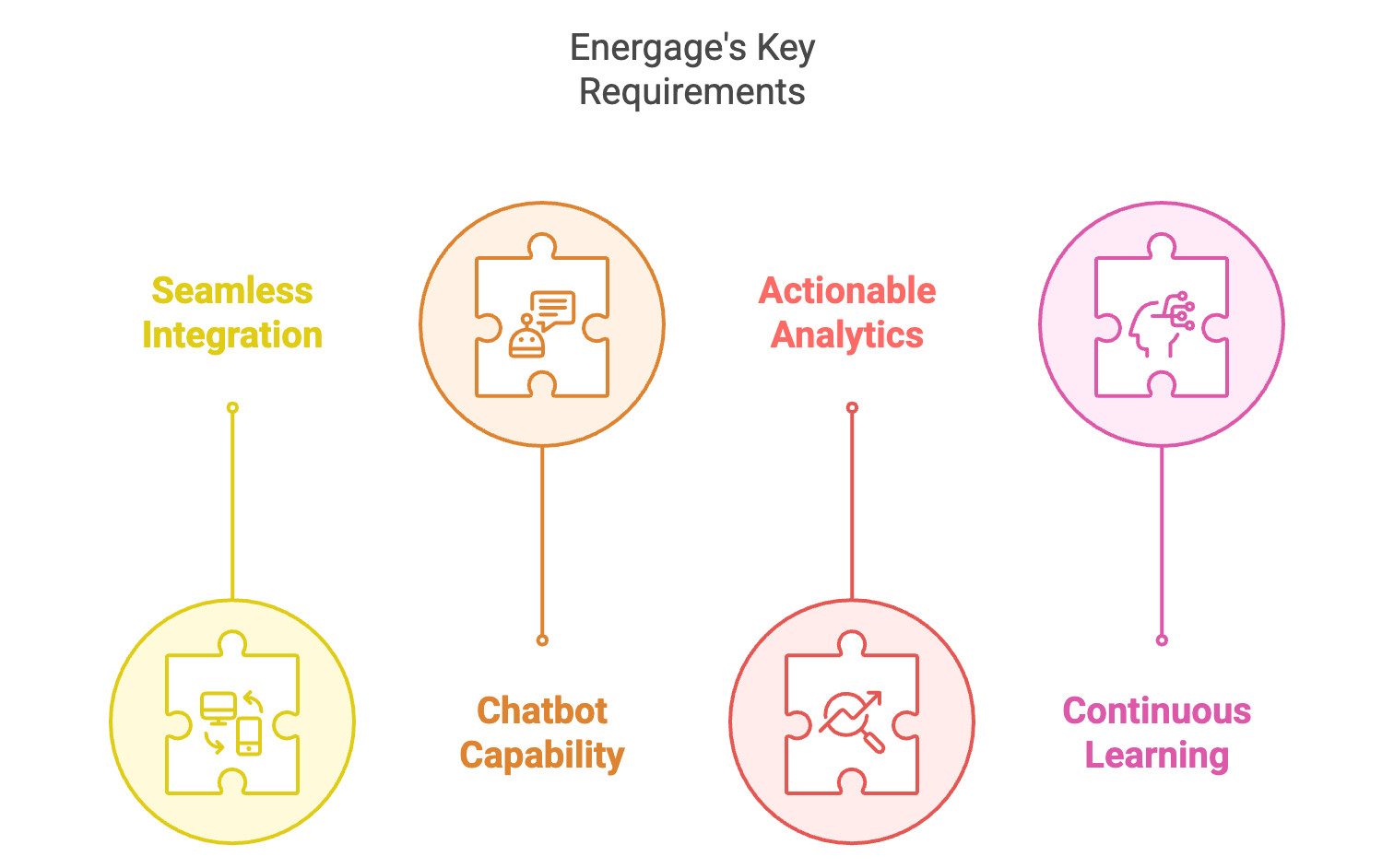
Rather than chasing flashy features or big vendors in the space, Anthony focused on these additional priorities:
- Who would be more hands on with his team where they wouldn’t feel like “just a number”?
- What would ROI look like and did it feel attainable?
- Was the technology enterprise grade ready?
- Were there additional use cases the team could roll out and leverage as future AI adoption needs would surface?
- What could give Energage and his support team an actual competitive advantage?
With these criteria in hand, it became easier to choose a solution that promised the quickest proof-of-value while meeting all requirements.
Curious to see what ROI may look like as a starting point? Try TheLoops ROI calculator.
Anthony’s advice for teams in a similar boat is to create a key requirements spreadsheet to measure and stack rank all vendors you’re evaluating and involve other departments within your company. Don’t be swayed by big tech or get shiny object syndrome. Stay objective and look for the best partner overall. You may also need to rework the ROI 1-2 times to land on a calculation that feels realistic vs pie in the sky. This is normal and to be expected.
On Preparing the Team and Building Correct Mindset
With the use case selected and integration under way, preparing the team for adoption became a competing priority.
We have often shared that introducing an AI Agent Copilot or any AI Agent isn’t just a technical project; it’s a behavioral change. However, no one really believes this until they see it themselves.
Energage tackled this head-on by:
- Seeding conversations early. Long before formal rollout, they hosted sessions on what AI Agent Copilot was and how agents would benefit from this more mature version of AI compared to the homegrown solution of Chat-GPT and Grammarly.
- Framing AI as augmentation. Every discussion with the team emphasized, “AI is meant to enhance you, not replace you,” easing fears of job loss thus improving adoption.
- Piloting with champions. Similar to TheLoops customer Gainsight and others, Energage worked closely with a cohort of team leads to test initial workflows, give feedback, and build confidence in Copilot’s capabilities before rolling it our broader.
- Gamifying engagement. A “feedback contest” continues to encourage agents to share observations, provide the Copilot with learning and move the needle higher on accuracy, which not only boosted usage but created a strong sense of partnership (AI and Agents, not AI or).
This incremental, people-first approach ensured that when Copilot launched, agents were more than ready—they were invested in its success.
Knowledge & Data Readiness: Avoiding Garbage In, Garbage Out
No AI project succeeds without clean, connected data. Yet being honest about the state of your data and the need to tackle it immediately can feel daunting.
In this short clip, Anthony shares about what to do first when your KB isn’t up to par — and why “eating the elephant one bite at a time” is more than just a saying.
Energage does have a customer education and enablement teams therefore Support quickly established some parameters for working closer together to improve the data in a swift but practical manner.
- Audit & alignment. They reviewed SOPs, KBs, and training docs to remove stale or conflicting content.
- Rapid update cycles. Bi-weekly sprints tackled the highest-impact content first, improving the AI’s ability to surface relevant answers.
- Unified structure. Internal and external documentation pipelines were streamlined to ensure Copilot pulled from a consistent, trusted source.
As Anthony puts it, “Once everyone’s on the same page, it’s a lot easier to move forward—because if AI can’t find or interpret our content, neither can the agents.”
His takeaway for others in this boat: don’t get discouraged. Put your arms around the reality of being reliant on too much tribal knowledge or irrelevant data. You’d be surprised how that first step, acknowledging whats needs to change, can allow the tactical process to move forward with a lot less friction.
The Bonus Value His Team Got From AI Agent Copilot
AI Agent Copilot’s summaries and faster knowledge retrieval were expected to deliver big wins. What truly surprised Anthony, however, was how much the agents came to rely on—and celebrate—the “Write/Reply” feature, which delivered:
- Polished, on‑brand replies: Agents—especially those for whom English is a second language—now draft messages with confidence in tone, grammar, and clarity.
- Instant insights & faster first replies: Relevant answers surface right in their workflow, cutting search delays and speeding up responses.
- Accelerated training lift: New hires onboard more quickly by studying Copilot’s suggested examples.
These unexpected boosts—in both employee experience and customer experience—have laid the groundwork for Energage’s next leap: rolling out an AI Autopilot capability, where AI will help with customer self-service. Thanks to the momentum from their Copilot pilot and the foundational KB improvements that followed, they’re perfectly positioned to expand seamlessly and sustainably.
Measuring ROI & Demonstrating Quick Wins
As we wind this blog down, we want to touch on one of the most important aspects to adopting AI: executive buy-in. ROI wasn’t a “nice to have”, it was a necessity and here’s who Anthony started to quantify it and make his business case.
First, he worked out three scenarios for the business: grow with headcount and no AI, grow with less headcount and AI or pursue AI only.
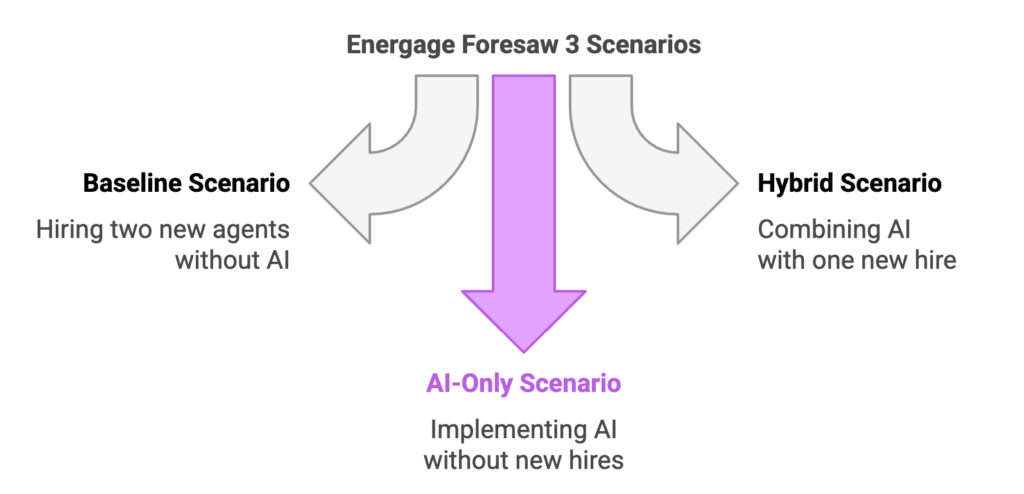
Once those numbers were factored, it was obvious that the AI only route using Copilot could deliver the equivalent uplift of a additional hires—at a fraction of the cost and ramp time. This would not only help all of the existing agents, but set them up for additional benefits to future hires. Here’s what else he factored into the ROI equation:
- Efficiency gains: Average handle time drops as agents used Copilot’s “Recommend Response” and “Summarize Case” features.
- Coverage improvements: AI-flagged knowledge gaps prompted targeted KB creation and updates.
- Adoption rates: The gamified feedback contest spiked usage within a week, anchoring the behavior change they needed.
Rather than defending a rigid “20% time savings” claim, he iterated the assumptions until everyone agreed on a baseline that felt both ambitious and achievable. That collaborative modeling built trust long before a single dollar was spent.
This exercise also inspired him to look at how they would uncover new metrics and calculate value. Beyond traditional CSAT and handle time metrics, Anthony and Energage now also track:
- Prompt adoption rate. How often are agents using Copilot features?
- Content-flag frequency. Which KB articles keep getting flagged for updates?
- Write/Reply confidence. A qualitative pulse—agents’ own rating of how much Copilot helps their tone and clarity.
These newer metrics surface early signals of value and guide the next round of improvements.
Setting Realistic Expectations
AI isn’t a flip-the-switch solution—it’s an ongoing journey. Like a new hire, AI needs time to train, adjust, and learn as it adapts to your team, data structure and discovers how to provide the most value:
- Usage won’t be 100% on day one. Both agents and the AI itself need a learning curve.
- Iterate, then iterate again. Value will grow as prompts, data and behaviors coalesce.
- Understand the pitfalls. What are the blockers for your team and how to overcome them.
- Celebrate small wins. Early adoption on one workflow paves the way for broader roll-out.
What matters most is momentum—each incremental improvement compounds into real strategic advantage.
Quick Recap: Turning Lessons into AI Action
Energage’s journey offers a clear roadmap for CX leaders ready to implement AI thoughtfully:
- Start with one use case. Focus on a high-impact workflow—like response drafting—to prove value quickly.
- Involve your agents early. Pilot with champions, gather feedback, and make adoption a team effort.
- Prioritize knowledge quality. Audit and streamline content so AI suggestions are accurate from day one.
- Model ROI collaboratively. Use calculators as a starting point, then refine with finance to gain alignment.
- Lead with people, not tech. Reinforce AI as a tool for empowerment, not replacement—and celebrate early wins.
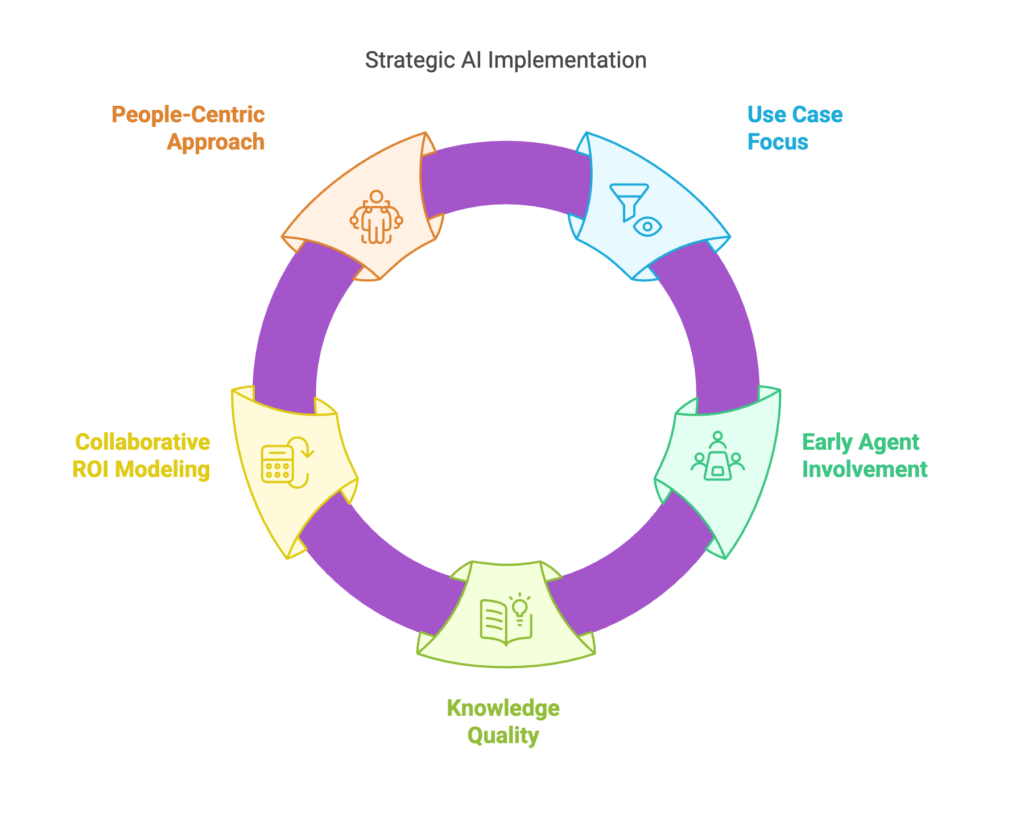
Conclusion
As Energage’s journey shows, successful AI implementation in CX is about finding the right balance of people, process, and technology. By rigorously defining business objectives, preparing knowledge base, and modeling clear ROI scenarios—from adding headcount to a hybrid approach to a pure AI solution—Anthony led the charge to set AI Agent Copilot as a competitive advantage. Their methodical, people-first strategy turned experimentation into empowerment, and early lessons into lasting value.
Ready to explore this for your team? Schedule a quick call here.