To fully understand how vital a semantic layer is to powering productive and relevant CX data experiences, we first need to acknowledge the limitations of Large Language Models (LLMs) and how they can be made more effective by introducing a semantic layer into the data stack (sitting in-between the LLM and the database).
Let’s first start by looking at the role LLMs play in the CX landscape.
LLMs comprehend the intent of their user then respond. Semantic layers ensure queries are correct. Together, they can answer questions with a company’s context in mind. And context is crucial in relation to improving the CX experience.
The Rise of LLMs
Large Language Models (LLMs) are a type of AI algorithm that use deep learning techniques and massively large data sets to understand, summarize, generate, and predict new content. The term generative AI is closely connected with LLMs, as they have been specifically architected to help generate text-based content. To put it simply, LLMs are next-word prediction engines.
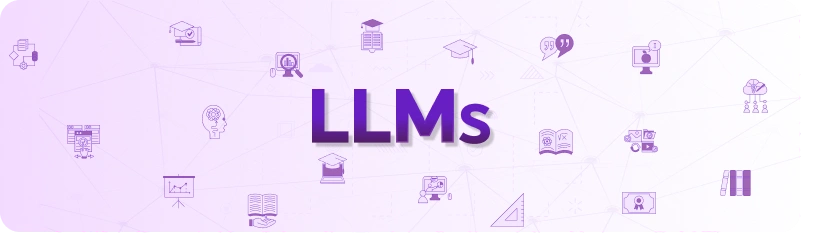
LLMs take the data consumption layer to the next level with AI-powered data experiences ranging from chatbots answering questions about your business data to AI agents taking actions based on signals and anomalies in data. They dramatically expand the volumes of data used for training and inference, providing a massive increase in the capabilities of the AI model.
You have all heard of OpenAI’s ChatGPT and Google’s Bard. When ChatGPT launched last year, it made the idea that generative AI could be used by companies and consumers to automate tasks, help with creative ideas, and even code software mainstream. While many AI tools are used in the workplace, none have created buzz quite like ChatGPT which has democratized AI beyond the realm of data scientists and specialized companies.
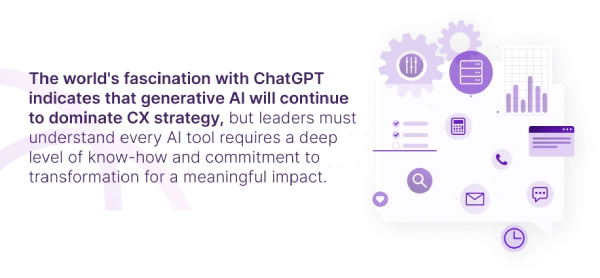
LEADER SPOTLIGHT: “AI will empower support leaders to create strategic support which is data-driven, allowing them to focus on building great experiences and culture. Using data insights to drive conversations with Success, Sales, and Product teams is now easy to achieve with AI. In the past, leaders didn’t have the luxury of building their data models and dashboards to ask complex questions. Now with AI, it’s all possible.
Margaret Lawrence Rosas – Customer Experience Executive , (Ex-Google and Looker)
The Limitations of LLMs
LLMs are indeed a step change, but inevitably, as with every technology, there are teething problems and drawbacks. LLMs hallucinate – the garbage in, garbage out issue. If a LLM is given biased or incomplete data, then the response it gives could be unreliable or plain wrong. Data analysts refer to this as hallucinations because responses can be so far off track. Remember, LLMs are predicting the next word based on what they’ve seen so far — it’s a statistical estimate and nothing more.
To operate correctly and execute trustworthy actions, a Large Language Model needs semantics about the outcome it is supposed to generate; it must also take into account the entities and entity-relationships of the domain. To do this it needs context from a semantic layer.
Semantic Layer Fixes LLM Hallucinations
Wikipedia describes the semantic layer as, “a business representation of data that lets users interact with data assets using business terms such as product, customer, or revenue to offer a unified, consolidated view of data across the organization.”
The semantic layer organizes data into meaningful business definitions and then allows for querying—rather than querying the database directly. The querying application is equally important as that of definitions because it enforces LLMs to query data through the semantic layer, ensuring the correctness of the queries and returned data. With that, the semantic layer becomes a solution for the LLM hallucination problem.
Zero-Shot and Validation
With generative AI, and in particular the hallucination issue, there is now more discussion around validation because LLMs are trained on world data, not enterprise data. This is why context in each of these models becomes really critical. If you follow the paradigm of how machine learning and model training has evolved, initially all the predictive algorithms used to be trained on data that is enterprise specific. But now, because you’re using a public model, it is being trained on data outside of your enterprise, which will result in returns that are not even part of your domain. This kind of prediction is called zero-shot prediction.
For zero-shot to work, you need to give it a lot of context. Context around what you’re expecting from the prediction, how you’re expecting it, and what format you’re expecting it in. By using terminology that is very specific to your interface, it will force the model to be written in an output that is very specific to what you’re asking for. Usually the first iteration is not good and necessitates numerous rounds of querying: a time consuming process.
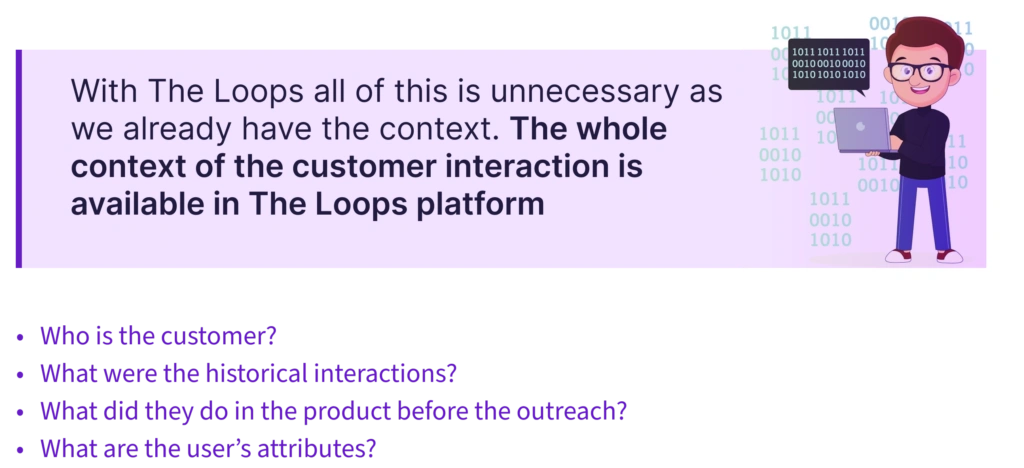
If you have this context at design time, you can provide whatever context you want to the LLM and drive a predictive engagement both for the user and agent at the point of contact. For example, when a user of a financial application faces an issue while making transactions, passing the context of the user journey to an in-product chatbot could not only help identify the right content but also personalize the content based on the usage patterns of the user’s session.
TheLoops helps bring the context together to help achieve hyper-personalization at scale with LLM in product.
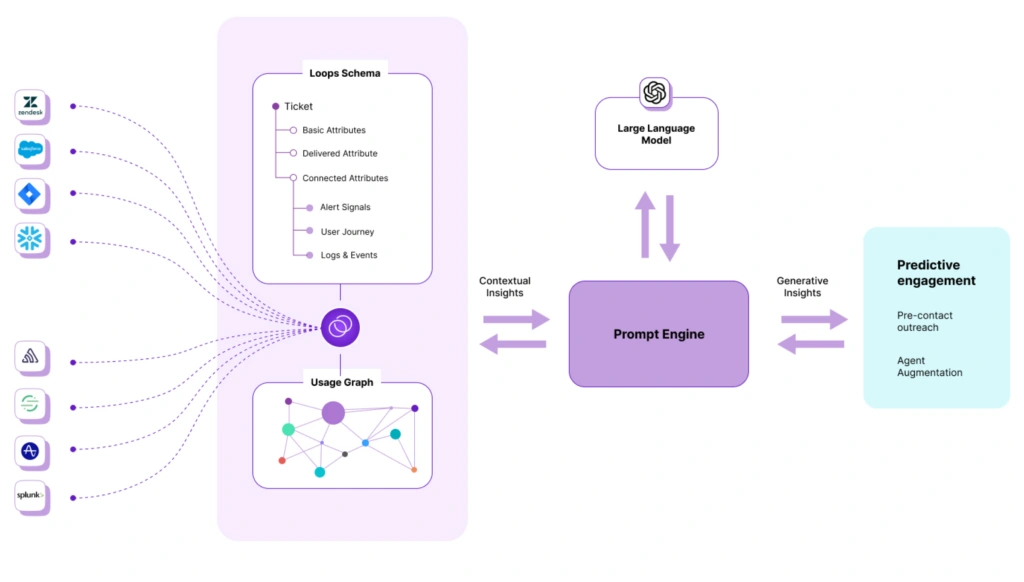
AI-powered Semantics Can Change the Dynamics of the CX Experience
The semantic layer provides CX professionals with easy access to the data needed for their specific roles and tasks. The semantic layer is the representation of data that helps different business end-users discover and access the right data efficiently, effectively, and effortlessly using common business terms.
It maps complex data relationships into easy-to-understand business terms to deliver a unified, consolidated view of data across the organization. This enables teams to work transparently and cooperatively with the same information and goals.
The semantic layer delivers data insights discovery and usability across the whole enterprise, with each business user empowered to use the terminology and tools that are specific to their role i.e. structured content with an intelligence shaped by semantics.
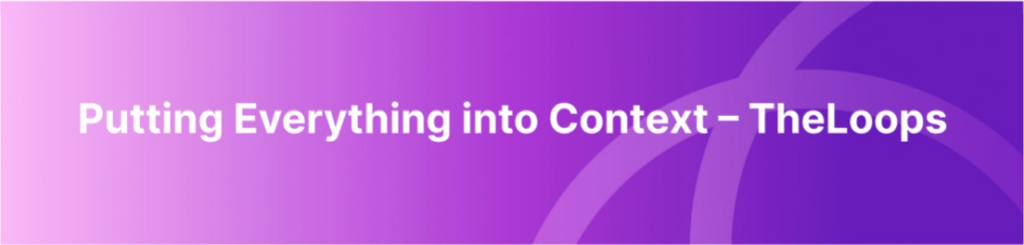
The technology benefits and CX potential discussed here are worthless without context–and again, context is something LLMs lack on their own. TheLoops is ideal for generative AI as we provide context and predictive capabilities to empower companies with a rich and informative CX data experience.
Embracing generative AI for CX is an essential step to delivering predictive engagement which provides exceptional and tailored experiences, improving operational efficiency and fostering innovation. By leveraging generative AI, businesses can achieve hyper-personalization which unlocks new levels of customer satisfaction, loyalty, and competitive advantage in the ever-evolving CX landscape.
LEADER SPOTLIGHT: “I truly think that this is where companies need to go, especially with the coming of GenAI. You can create a secure digital experience right within your product/platform/web app. If you have that, why would you have to leave the application for assistance, purchase, renew, etc? Everything should be done right through there, without having to leave it to go to a support portal or anywhere else. Plus, your customers are already authenticated, so you can further personalize the experience. To me, it makes all the sense in the world.” Ben Saitz, Chief Customer Officer, Netskope
TheLoops provides contextual awareness on all aspects of the customer journey. By analyzing data from Zendesk, Slack, Amplitude, Jira, PagerDuty, or Salesforce, TheLoops presents insights for in-product support and recommendations to guide agents. Agents must be fully aware of what issues the customer is experiencing and must have access to data in a single pane. With a complete picture, support agents can troubleshoot problems on-the-fly as well as identify unserved needs of their customer.
With contextual awareness, first line agents and support managers are able to take CX to the next level. Contextual awareness is how businesses can deliver modern support. Agents transform from being reactive to growth oriented. A customer experience that converts tickets from support issues to opportunities for growing the lifetime value of your customers.
Organizations must contextualize data from a wide array of sources and intelligently resurface it to agents, customer success managers, leaders, engineers, to help them understand exactly where the customer is in their journey. However, you don’t want to bombard agents and customer success managers with reams of information, it has to be tailored, recommended data. Automated support operations not only resolve issues on the frontline but completely closes the loop with the customer.
Final Words
The combination of LLMs, semantic layers and TheLoops enable a new generation of AI-powered CX data experiences. LLMs and the semantic layer combine high level intelligence with context of the business that allows deep and accurate question/answering. Companies that have the right data integration layers will ultimately be the winners in the generative AI revolution.
Ready to explore TheLoops for you and your team? Request a demo here.