One of the biggest challenges Support leaders face is deciding where and how to begin AI implementation for CX operations. With new providers popping up in the market everyday — it’s natural to get overwhelmed with decoding next steps.
To help provide insights and best practices, we interviewed Matt Dunn, a Support leader and Director who has rolled out AI for his Support team multiple times throughout the past 5-6 years. In fact, long before the GenAI rush, Matt and his team invested 9 months alone to train AI models and deploy their first use case with another AI vendor.
More recently, he and his team were early adopters of TheLoops AI, rolling out a streamlined, turnkey plan in under 90 days.
Given his experience with multiple approaches to AI implementation, we wanted Matt to share how Customer Support leader’s can think about this process and avoid pitfalls. If you’re evaluating AI for CX for your organization, read below for Matt’s advice on how to progress and improve your CX operations with the right strategy in place.
1. Start Small, Think Big, Automate Immediately
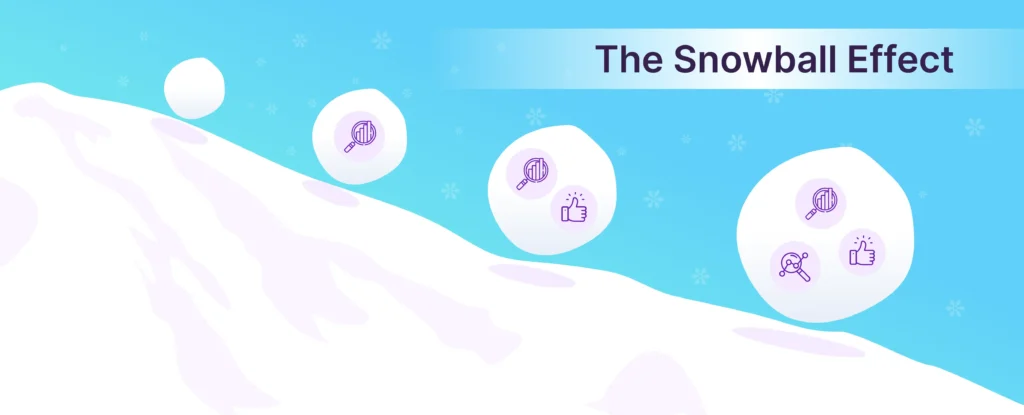
One of the hurdles Support leaders face is determining where to begin with AI implementation.
Matt advocates for a practical approach, suggesting that leaders start by identifying routine tasks that are both time-consuming and manual. He explains:
“I was thinking about this, and I think that the real first starting point for me was in just thinking about things that I sort of semi-regularly had to do. Often times, it was pulling data together. For example, I might need to create an at-risk list of customers or something like that. I’d want to pull in customer data and support ticket data, do a review of where they’re at, and analyze the kind of problems they’d been running into.”
While companies often feel pressure to focus on customer facing AI first, Matt suggests looking at AI for CX processes that are best suited for automation and insights.
“As a manager, I had a lot of things like that would come up semi-regularly which required a lot of variance. It might not always be the exact same initiative or task that came up each time, but accessing data was always a manual process. I’d have to go and pull some out of Zendesk, pull some data out Salesforce and some from Jira, then end up throwing it all into a spreadsheet and trying to make sense of it all. It used to take hours to days of my time.“
One area Matt implemented to right away to replace this was proactive backlog management. “Within days, TheLoops enabled a dashboard for me to see high impacted tickets and customers to focus on.”
The advantage of this strategy lies in its scalability and momentum-building potential longterm. As Matt specifies, “Once you identify that first area and you start, the ball starts rolling down, and then it’s kind of like, okay, what else can I do?”
A snowball effect is crucial. It helps teams to see immediate benefits while gradually expanding AI use cases across other areas of Support operations. And automating manual processes while also gathering immediate, contextualized insights helps builds confidence. It generates buy-in from stakeholders, and creates additional opportunities for AI integration. For Matt and his team, their next use case was AI Agent Copilot.
2. Leverage A Platform To Uncover AI’s True Potential
Another issue that comes with AI adoption is how many organizations fall into the trap of collecting multiple point solutions for each new problem they encounter. Matt advises against this approach, advocating instead for leveraging one platform with extensibility.
He explains, “By stacking point solutions, you’re just duplicating effort, money, and time. The advantage of a platform like TheLoops is that once you’ve done a lot of that integration work and you get those first use cases taken care of, then when you get that momentum going, and the next important use case you identify becomes so much easier and quicker to do and to solve. You’ve got things you can combine together rather than just having completely separate things again.”
Instead of looking at each issue as a different puzzle requiring a unique solution, Matt suggests that Support leaders step back and consider the bigger picture. He emphasizes:
“This is a really important topic because I think a lot of people think about things as individual problems or pain points. I think it’s worth thinking about what systems and sources of data you have that are common for solving all of these types of problems.”
By integrating various data sources and workflows into a comprehensive platform, Support leaders can ensure that their AI strategies grow seamlessly as needs or even the tech stack changes. This not only reduces redundancy and controls costs but also simplifies the implementation of additional AI use cases as they arise.
For another example on best practices of AI implementation, read how Bloomreach reinvented Support with TheLoops AI.
3. Share Your Immediate Wins and Real-Time Data
To gain buy-in from both Support and other departments like Success or Product, Matt stresses the importance of demonstrating immediate wins and providing valuable insights.
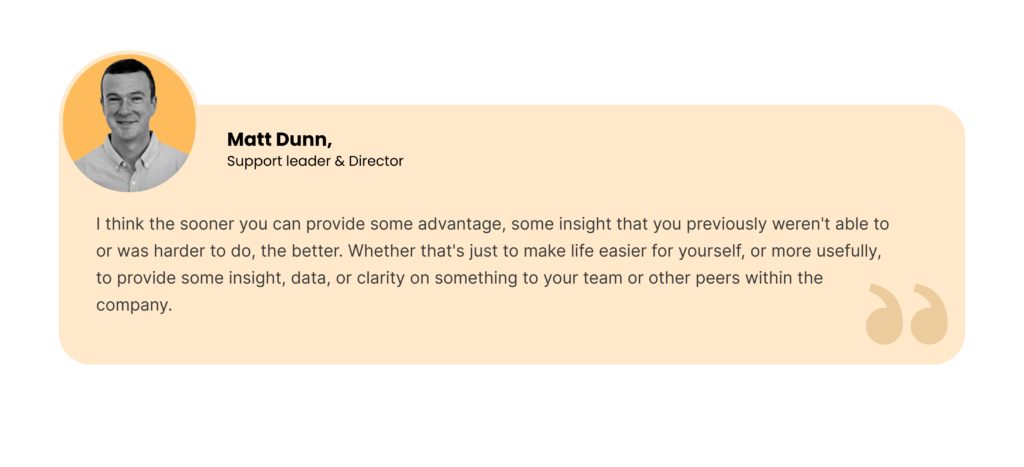
By showcasing how AI can solve existing pain points or uncover new customer data that’s often hidden and unstructured, Support leaders can build trust in AI’s validity. Matt illustrates this with a practical example:
“We talked about that use case of providing information to our product team around a feature request – who are the customers who’ve asked for this? What are the account sizes? How important is it to each of these customers? Being able to provide that clarity and insight to another team in the business on an ongoing basis is a real win.”
This example underscores how AI can bridge gaps between departments, fostering collaboration and driving data-driven decision making. It’s not just about improving Support operations; it’s about elevating the entire post-sales ecosystem and aligning around NRR as a team.
Matt further emphasizes the importance of these early wins in catalyzing broader adoption:
“So, with implementation, I think it’s about identifying and getting to that point where you’ve got that first ‘Aha!’ moment – ‘Okay, this is what I can now provide that we weren’t able to easily provide before.’ People then start to sit up and take notice a little bit and say, ‘Okay, this really helps me answer the question: what else can we do?'”
4. On Managing Expectations and Encouraging Patience
Just as a new hire needs time to adapt to their role, learn processes, and become fully productive, Matt reminds leaders that AI models also need a period of adjustment and learning. This ramp-up phase is essential for the AI to understand the unique nuances of an organization’s data, processes, and customer interactions.
Drawing on his own experience, Matt states: “Part of how people get up to speed is repetition. The more often you do something, the more you repeat something, the better you get at it, the more quickly you get to the point where you solve the task or whatever it is you’re trying to do, and with AI, it’s exactly the same.”
AI requires feedback, learning and a timeline to improve. This is especially important for Support leaders to remember: don’t expect immediate perfection from AI software. Just as you provide feedback and coaching to new team members, AI needs a similar “human-in-the-loop” approach during its initial implementation and ongoing refinement.
Matt further elaborates on the balance required in this process:
“You could spend a long time in implementation and training and so on to try and get to that extra tiny bit more accuracy or confidence, but you’ve got to find that balance.”
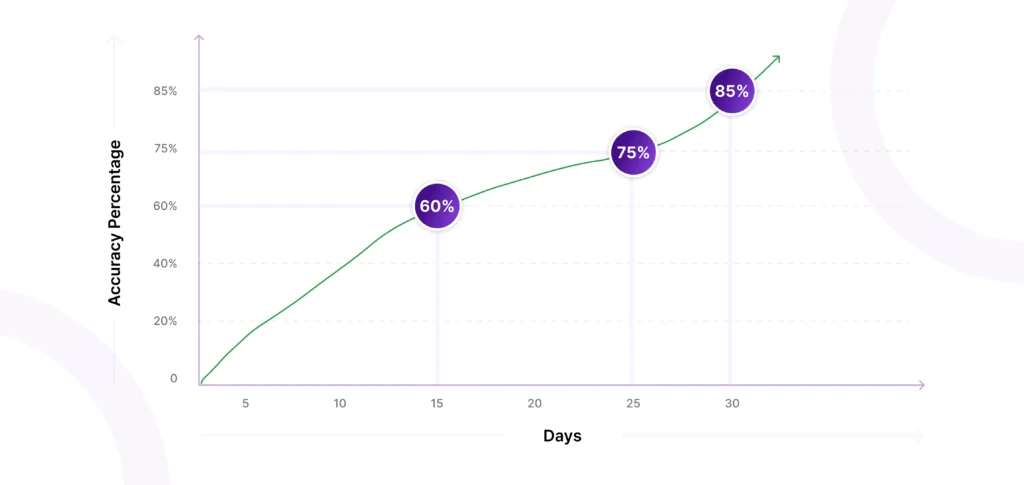
While it’s important to invest time in properly training and implementing AI systems, it’s equally important not to get caught in an endless cycle of perfectionism. The goal is to reach a point where the AI is sufficiently accurate and reliable to provide value, while understanding that it will continue to improve over time with more data and interactions.
5. Shifting Metrics and Mindset: Subtract Manual, Add Improvements
As organizations adopt AI in Customer Support, traditional metrics specific to operations may need to be reevaluated. While many leaders focus on specific targets, such as improving Mean Time to Resolution (MTTR) by a certain percentage, Matt suggests a more nuanced approach.
“I always think about it more as what are the things that are preventing you from doing that right now, or preventing you from increasing your MTTR,” he explains.
Instead of fixating on numerical, surface level goals, Matt recommends identifying the time sinks and inefficiencies in current processes. By overcoming these inefficiencies with the help of AI, improvements in metrics like MTTR will naturally follow. This shift in mindset from purely metric-driven goals to process improvement and efficiency can lead to more sustainable and meaningful outcomes as well as cost savings. For what it’s worth, Matt and his team were able to save $480,000 with TheLoops AI in place.
Adopting AI as a Journey
Integrating AI in customer support is not a destination but a journey. It requires patience, strategic thinking, and a willingness to learn and adapt.
By viewing AI as a strategic partner for Customer Support — one that needs time to learn and grow, just like any new team member or consultant— organizations can position themselves at the forefront of customer service innovation.
As technology and customer preferences continue to change, those who have laid a solid foundation for AI adoption will be best equipped to leverage new updates, staying ahead in an increasingly competitive economy.
Still on the fence regarding AI implementation? Watch this fireside chat we did with Ravi Bulusu, CTO and CO-Founder of TheLoops AI. He answers all the questions you might have regarding AI implementation for CX operations.