AI in CX is a hot topic thanks to Generative AI. But as we shared last month in our “Beyond The Bot” segment, AI in CX is not just one thing. AI is actually a multiple of parts including Topic Modeling, Scoring, Prediction as well as Generative AI.
In this blog, we are going to give light to Topic Modeling and it’s applicable use-cases in CX. When evaluating your next AI solution and partner, this is what you should be looking for–solutions or platforms that offering Topic modeling. Here’s why.
CX data provides a wealth of information for companies.
Take a moment to stop and think about all of the attributes that occur within support requests: verbal and textual conversations, phone calls, emotional exchanges, time delays, and other types of data are generated. While marketing and sales teams vie for the customer’s time and engagement, Support teams obtain the customer’s attention loud and clear. And each customer interaction, good and bad, leaves a digital footprint rich in detail.
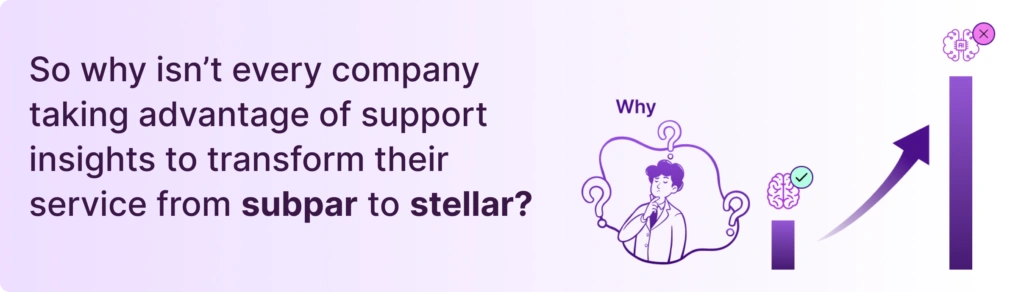
Up until now, the ability to understand these on a deeper, actionable level, much less convert the information support data provided into opportunities, has been manual, time consuming and cumbersome. Someone on the team had to scan and read each and every ticket and interaction to obtain a full picture as to what was happening. That was, until AI came along.
Now, the question for companies up-leveling their CX, by way of AI, is how does everyone across the organization, from top down, benefit from support data? How can we use AI to fix the broken model of manual analysis, tagging and labeling which is inefficient and doesn’t solve the customers’ issues any faster? How does this help drive automation downstream from these generated insights?
One idea is topic modeling.
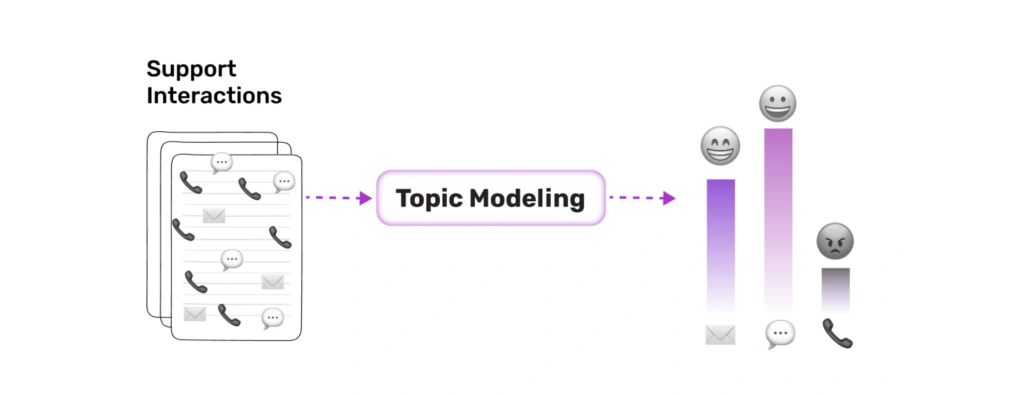
Topic modeling is a form of AI that enables CX teams (support, success, product and engineering) to move away from delayed understanding and instead, quickly identify prevalent themes customers are experiencing. In turn, this immediate analysis provides companies the opportunity to respond faster and deliver highly personalized, relevant, improved experiences to customers.
We’ll dig into topic analysis a little deeper but before we do, we can’t emphasize this enough–there’s more than one type of AI available to customer facing teams. In fact, here’s a list of options available.
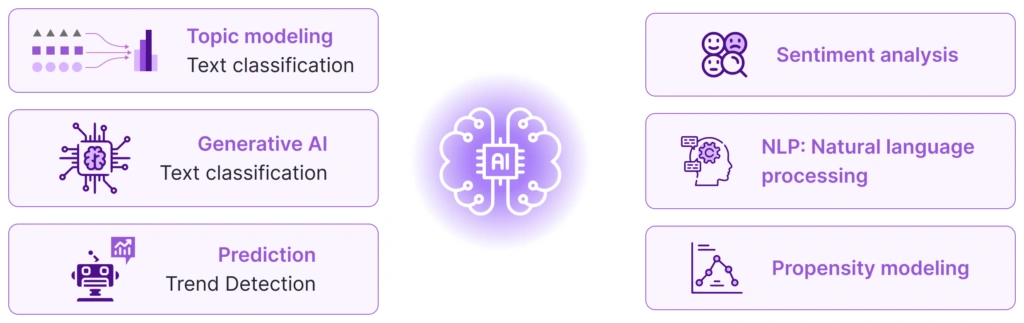
As you can see, AI is more vast and varied than the headlines reflect. With that in mind, let’s explore the concept of topic modeling and how it’s beneficial for the customer’s experience. The answer may surprise you.
What Is Topic Modeling?
At its core, topic modeling helps businesses identify common themes or topics within large sets of customer feedback data. How exactly does topic modeling work? And why is it recommended for CX teams? It can also be looked upon as creating categories which is done manually and changed every 3-6 months.
The clue also lies in its name. Topic modeling is a technique that uses NLP (natural language processing) and machine learning to find latent topics in text data. Latent means these topics are hidden and concealed from an end user and can only be uncovered by deeper, statistical analysis hence the previous need for a data scientist to synthesize this information. Various algorithms have been used for topic modeling, including Probabilistic Latent Semantic Analysis (PLSA), Latent Dirichlet Allocation (LDA), and Non-negative Matrix Factorization (NMF).
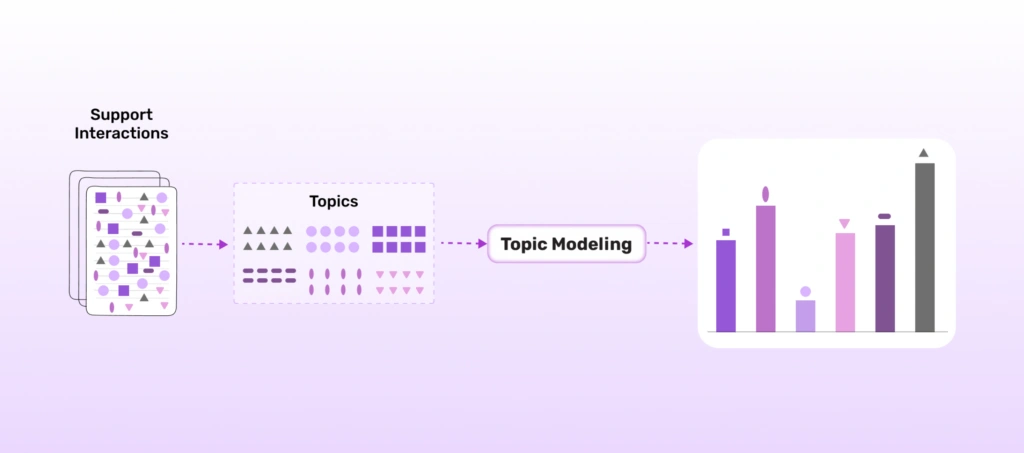
Remember those customer interactions from the opener of this piece? The emails, chats, even calls? Topic modeling analyzes that large collection of text then identifies patterns of co-occurring words or phrases that suggest the presence of underlying themes or topics.
Choosing the right algorithm for topic modeling depends on the specific task, the size and complexity of the dataset, and the desired level of interpretability. Researchers and practitioners often experiment with different algorithms, supervised vs unsupervised, and hyper parameters to find the best approach for their particular application.
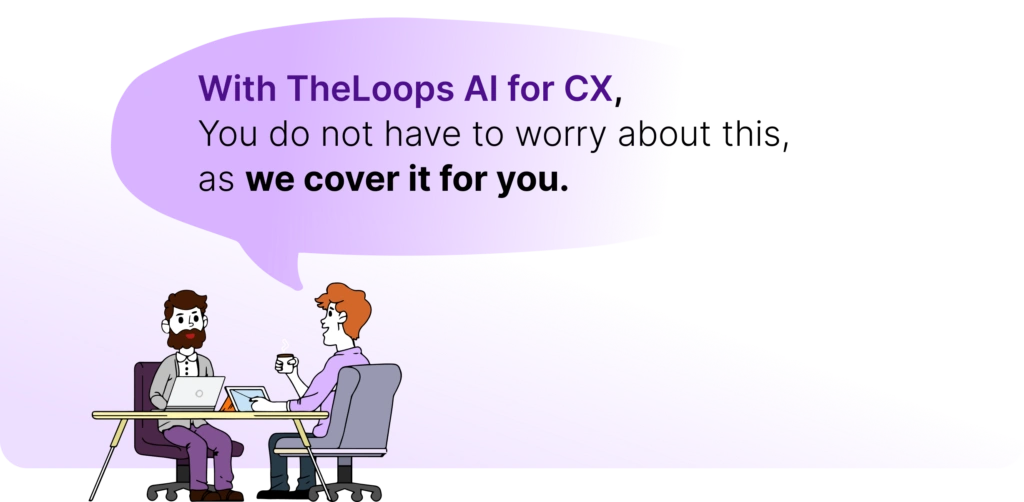
As a result of topic modeling, support teams, managers, product marketers, customer success managers and even the C-Suite can better understand customers’ needs and pain points.
As simple as this may sound, topic modeling has transformative benefits.
- It can realign decision-making and prioritization around product development, business operations, customer support response, training and processes, and even marketing.
- It can help with auto-classification and routing.
- Lastly, it can find clusters within your data that you are not aware of.
The best part about it is that companies no longer need a data scientist, someone who specializes in statistical analyses and interpretation, to implement topic modeling.
Thanks to the rise of AI, there are a variety of user-friendly tools and platforms, such as TheLoops, that make topic modeling accessible for customer-facing teams with the click of a button.
Why Is Topic Modeling Important for CX Leaders?
When it comes to customer support and success, topic modeling is used across a variety of functions, including auto-classification and routing of tickets, product feedback analysis, content recommendations, and customer sentiment analysis.
All of these help drive business outcomes, which increase the overall efficiency and productivity of teams without requiring manual tagging and dumping the data in excel.
CX teams can spend anywhere up to 2-3 months doing this which is not at all efficient.
Topic modeling also helps you understand what customers are talking about when it comes to feature requests, how to classify them based on the topics highlighted – coupled with the sentiment that’s trending. With it, you can set up automations on topics for the product team re: the impact of new features released to market. Or classify tickets from a data-driven approach and see the impact within each cluster in terms of ARR and number of customers asking. You can even use this to see where your team is not performing based on the issues that are getting escalated. Taking it a step further, you can also use these topics to share updates on this in Asana, Productboard or Jira for your engineers.
With this overview in mind, here’s a specific example of how much more impactful your customer experience, support and success becomes with Topic Modeling.
Let’s use the services of a phone provider.
The provider has a Customer Support team, an Account Management team and an Engineering team.
On a monthly basis, Support receives a high volume of customer issues around “billing”–10,000 on average.
Without topic modeling, the entire team is at least able to deduce: our customers have a billing problem.
With topic modeling, here’s how specific they can get with hierarchical topic classification.
Under billing alone, you can quickly drill down, and learn about the specific subset of actions customers are trying to take:
- Are they creating an invoice?
- Deleting an invoice?
- The team can reflect: did our onboarding or knowledge base fail to guide them in doing this?
- Changing a payment method?
- Inquiring about a fee or pricing change?
- Can the account manager or support team jump in to minimize cancellation and churn?
- Were they billed twice?
- Why did this happen? Is it user error or is there a bug engineering needs to fix?
Without topic modeling, you are operating at the surface level.
Analytics will reflect a large grouping of customer issues around BILLING but you won’t have specifics or context as to which billing issues are occurring and how often. When stuck in silos and hidden from your eye, you won’t see what the impact of this is paired with contextual data from different systems.
With topic modeling, you are able to drill down deeper and uncover issues then involve the appropriate cross-functional teams to help you resolve them from occurring in the future.
Best of all? AI can gather all of this data, analyze it and report it back to you in real-time.
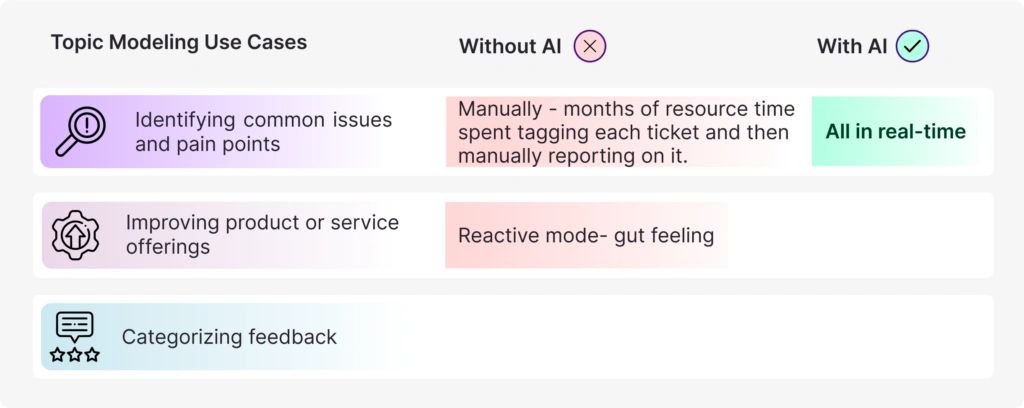
Our CTO+Co-Founder, Ravi Bulusu, who holds 26+ patents in machine learning, is a big advocate for Topic Modeling in CX because, “you can uncover this information right out of the box without requiring too much configuration or training.”
In a follow up piece to this, we’ll explore what training means when it comes to AI, algorithms and models. We’ll also share more on the history of topic modeling and how it’s different from a technique called clustering.
Curious to hear more about how TheLoops uses Topic Modeling? Book a demo with our team.